Dynamic data processing: Recursive least-squares
Synopsis
This book is a follow-up on Adjustment theory. It extends the theory to the case of time-varying parameters with an emphasis on their recursive determination. Least-squares estimation will be the leading principle used. A least-squares solution is said to be recursive when the method of computation enables sequential, rather than batch, processing of the measurement data. The recursive equations enable the updating of parameter estimates for new observations without the need to store all past observations. Methods of recursive least-squares estimation are therefore particularly useful for applications in which the time-varying parameters need to be instantly determined. Important examples of such applications can be found in the fields of real-time kinematic positioning, navigation and guidance, or multivariate time series analysis. The goal of this book is therefore to convey the necessary knowledge to be able to process sequentially collected measurements for the purpose of estimating time-varying parameters.
When determining time-varying parameters from sequentially collected measurement data, one can discriminate between three types of estimation problems: filtering, prediction and smoothing. Filtering aims at the determination of current parameter values, while smoothing and prediction aim at the determination of respectively past and future parameter values. The emphasis in this book will be on recursive least-squares filtering. The theory is worked out for the important case of linear(ized) models. The measurement-update and time-update equations of recursive least-squares are discussed in detail. Models with sequentially collected data, but time-invariant parameters are treated first.
In this case only the measurement-update equations apply. State-space models for dynamic systems are discussed so as to include time-varying parameters. This includes their linearization and the construction of the state transition matrix. Elements from the theory of random functions are used to describe the propagation laws for linear dynamic systems. The theory is illustrated by means of many worked out examples. They are drawn from applications such as kinematic positioning, satellite orbit determination and inertial navigation.
Downloads
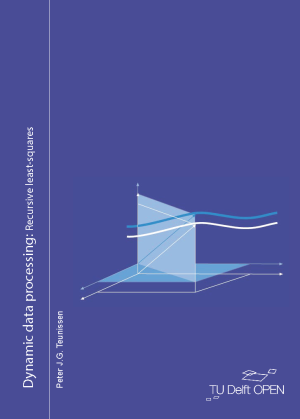